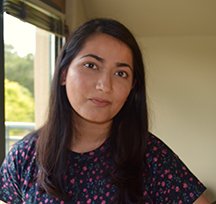
Speaker: Urvashi Khandelwal, Google AI
Title: Generalization through Memorization
Date: Friday. January 14, 2022 - 1:30 - 2:30 PM EST (North American Eastern Standard Time) via Zoom
Zoom Access: Zoom Link and reach out to Alex Taubman for the passcode.
Abstract: Neural language models (LMs) have become the workhorse of most natural language processing tasks and systems today. Yet, they are not perfect, and the most important challenge in improving them further is their inability to generalize consistently in a range of settings. In this talk, I describe my work on “Generalization through Memorization” -- exploiting the notion of similarity between examples by using data saved in an external memory and retrieving nearest neighbors from it. This approach improves existing LM and machine translation models in a range of settings, including both in- and out-of-domain generalization, without any added training costs. Beyond improving generalization, memorization also makes model predictions more interpretable.
Bio: Urvashi Khandelwal is a Research Scientist in the language team at Google AI. Prior to this, she was a PhD student in Computer Science at Stanford University, in the Stanford Natural Language Processing (NLP) Group, where she was advised by Professor Dan Jurafsky. She works at the intersection of NLP and machine learning, and is interested in building interpretable systems that can generalize in and adapt to a range of settings. Her research was recognized by a Microsoft Research Dissertation Grant in 2020.